Researchers at IISc are using novel technologies to add to existing materials and to understand material properties
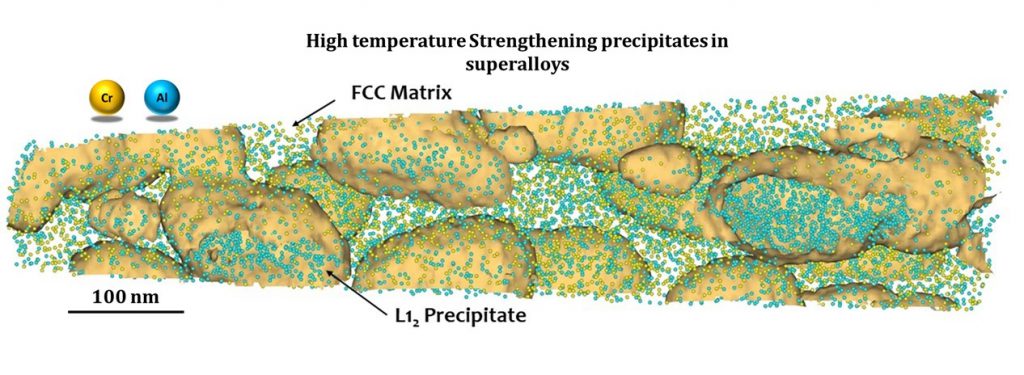
(Image: Nithin Baler)
About 2.5 million years ago, our ancestors discovered that striking two pieces of rock against each other produces rough edges and stone chips that could be used to break animal bones and scoop flesh off carcasses. And thus, the first stone tools were made. But it was no mean task to shape them, especially as they became more sophisticated over time. It was only around 12,000 years ago that humans started to make tools with metals that could be heated and moulded with relative ease. The first metal tools were made from shiny copper bits found on riverbeds. Years later, humans discovered that mixing copper with tin makes for stronger tools. This marked the dawn of the Bronze Age; humans had now learnt the art of metal-working and alloying. The Bronze Age was followed by the Iron Age, which lasted until about 2,000 years ago. Each of these new technologies transformed our lives and is a milestone in human history.
In the past few centuries, new materials have continued to shape the course of our progress. Thanks to modern materials like iron, steel, silicon, and plastics, descendants of hunter-gatherers now shop for groceries in supermarkets carrying smartphones and designer bags. The 21st century is again seeing a revolution, as researchers across the globe are in pursuit of novel materials for various applications using state-of-the-art technology.
Building new blends
Unlike in the past, the approach to developing new materials in the modern age is much more scientific. Among new materials, alloys are of particular interest because they find uses in areas as diverse as construction, transportation, defence, and energy. Researchers design new alloys by selecting elements and blending together based on their crystal structures and phase diagrams, according to Surendra Kumar Makineni, Assistant Professor at the Department of Materials Engineering, IISc. While the crystal structure describes the arrangement of atoms in a definite pattern, the phase diagrams are a graphical representation of the ‘phases’ or state of an alloy at different temperatures and compositions. The type of phases dictates alloy properties, and phase diagrams help researchers to find the compositions that yield desired properties.
Surendra’s group works on the synthesis of new alloys for structural and high-temperature applications, like superalloys for gas turbines. Gas turbines provide thrust to aircraft by igniting fuel within them; as a result, temperatures within it could be as high as 1500°C. “The higher the operating temperature, the higher the operation yield,” explains Surendra. Developing materials that can withstand high temperatures would enable us to improve the fuel efficiency – or how far the aircraft can travel per unit of fuel – of aircraft engines and reduce greenhouse gas emissions.
The 21st century is again seeing a revolution, as researchers across the globe are in pursuit of novel materials for various applications using state-of-the-art technology
Traditionally, nickel-based alloys have been used in turbines because they are stable at high temperatures owing to their unique two-phase structure. But they contain dense and less abundant elements which are heavy and expensive. Surendra’s lab is, therefore, working towards reducing dense elements without compromising the alloys’ high-temperature properties.
During his PhD at IISc, Surendra also helped develop a new class of low-density cobalt-based alloys. The advantage of cobalt is that it has a melting point that is higher than nickel by 40°C and can form a two-phase structure similar to that of nickel-based alloys, he points out. “We have shown that some of the [cobalt-based] alloys have better properties than nickel-based ones,” he says, making them an attractive option for building turbine components. His group is also exploring ways to increase their performance at higher temperatures by adding other elements.
Modelling material properties
In 2011, the then US President Barack Obama launched the Materials Genome Initiative (MGI), with an aim to accelerate the discovery and development of new materials at lower costs. This provided a major thrust to the field of computational materials sciences, according to Abhishek Singh, Professor at the Materials Research Centre, IISc.
Abhishek’s lab specialises in using machine learning tools to advance materials science and development. These tools help develop new materials faster, according to him. “When you search for a new material with traditional science, it will take you 20 to 30 years. Now, you can do it in one to two years for database development, and another six months of model development. Nowadays, there are many databases already [available]; so you could use them directly,” he says.
Machine learning is particularly useful for correlating material properties that are not connected through underlying theory, or connecting experimental data to material properties – an application Abhishek’s group has been exploring. They recently developed a model that predicts the hardness of a superalloy using scanning electron microscope (SEM) images. This would greatly reduce the time and cost required to experimentally develop the alloy and determine its hardness, which would enable experimentalists to test alloys for various applications easily.
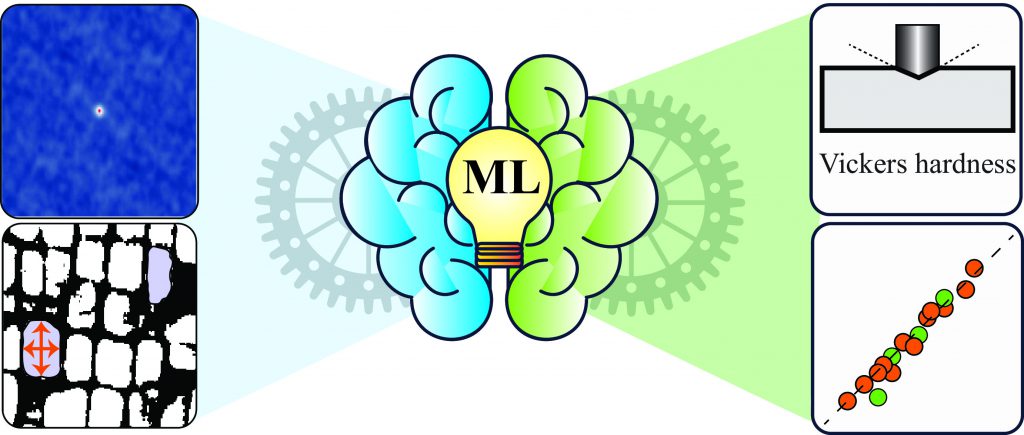
(Image: Abhishek Singh lab)
Abhishek and his group reduced SEM images to binary data, and processed this data using statistical tools to choose only those ‘features’ or image parameters that were sufficient to encapsulate the data accurately. These features, along with material composition and information regarding material processing, were used to generate a model that could predict the hardness of the material. They also developed a second model using geometrical attributes of the materials extracted from the SEM images and processing information and found that the hardness predicted by both these models was very similar. These models for predicting hardness can be extended to any material, says Abhishek. His lab now has ambitious plans for this in the near future. “[We are working on] an app [where] if you upload your SEM image and give the compositions of your alloys and some processing information, it will predict the hardness for you from these two approaches,” he spells out.
Little things matter
Often, designing materials for engineering applications requires researchers to understand how events at a molecular or crystal level contribute to the properties of the whole material, and this cannot be done using experimental methods. This gap can be bridged using a suitable modelling approach, according to Debiprosad Roy Mahapatra, Associate Professor at the Department of Aerospace Engineering, IISc. His group recently modelled material microstructures – material structures that appear at a small scale – to help predict mechanical and fracture-related properties of bulk materials.
Since metal surfaces appear uniform to the naked eye, it is easy to assume that they show similar properties along different directions; yet, when exposed to a load, cracks appear at specific locations and in different directions. It is often difficult to predict when and where they will initiate, and in which direction they will grow, but this information is hidden in material microstructures, says Debiprosad. SEM images of metals reveal crystals in different orientations – or ‘grains’ – and their boundaries; some of these crystals have defects, and these defects tend to arrange themselves along certain planes in the material. This can create excessive stresses in certain regions when loaded, leading to material failure along certain directions, he explains.
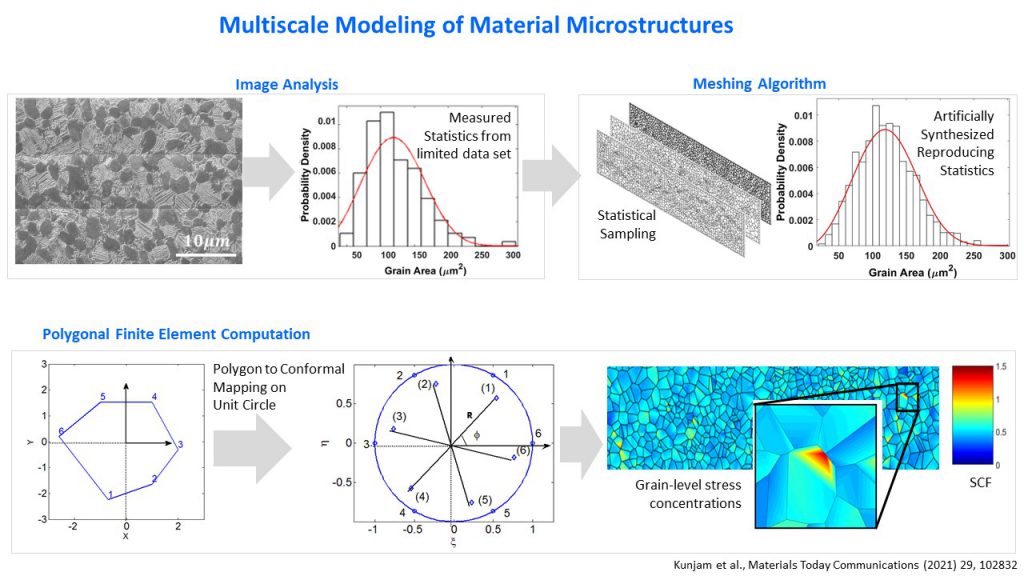
(Image: Mahapatra lab)
With the help of SEM images, Debiprosad and his team determined the probability distributions of certain parameters like grain size by analysing different regions of metals, like in titanium alloys used in aerospace applications. Using these, they synthetically created an artificial mesh that simulated the original image. They then used a mathematical technique – the polygonal finite element method – to model the grains and grain boundaries accurately in order to predict crucial properties of the material. They computed the stress concentrations at different regions of the mesh, and were able to predict where cracks are more likely to develop in the material. “Further work is in progress where we are linking this information to molecular dynamics. We hope to be able to predict molecular level fatigue nucleation sites from this information,” Debiprosad says.
‘Transition’ing to efficient energy
Lithium-ion batteries have wide-ranging applications in devices like mobile phones, and in electric vehicles. These batteries are powered by the flow of lithium ions from the negatively charged electrode (the anode, typically graphite) to the positively charged cathode through a liquid electrolyte. However, with this architecture, we are approaching the limits on the energy that can be stored per unit mass or volume of the cell – or its energy density – says Sai Gautam Gopalakrishnan, Assistant Professor at the Department of Materials Engineering, IISc. “If we can improve the energy density, we can use our batteries for longer; this could make our portable electronics last longer, and increase the driving range for electric vehicles,” he suggests. The promise of more efficient batteries drives his interest in exploring calcium-based batteries as possible alternatives to lithium-based ones.
Calcium batteries offer several advantages as compared to lithium ones; unlike the latter, which have graphite based anodes, calcium batteries can use metal anodes. “Graphite occupies a lot of space, and it’s dead-weight; it doesn’t contribute to the electrochemical process. It’s just a place to store lithium, because metallic lithium is very reactive,” Sai explains. He argues that replacing the anode with a metal would improve the energy density of the cell. Calcium is also much more abundant as compared to lithium, and has similar electrochemical properties. Calcium, therefore, promises to be an attractive replacement for lithium in batteries.
Sai and his team recently adopted a computational approach to identify candidates to serve as cathodes in calcium batteries. “The approach that we take is what I call the Iron Man approach; Iron Man basically runs simulations on J.A.R.V.I.S., which is his AI, and he comes up with [new] things. So that’s the approach we want to take. We don’t want to necessarily go into the lab to find a new candidate, but run calculations and simulations and be able to robustly predict new candidates,” says Sai. This helps reduce the time to innovate, and allows researchers to test a large number of materials, he adds.
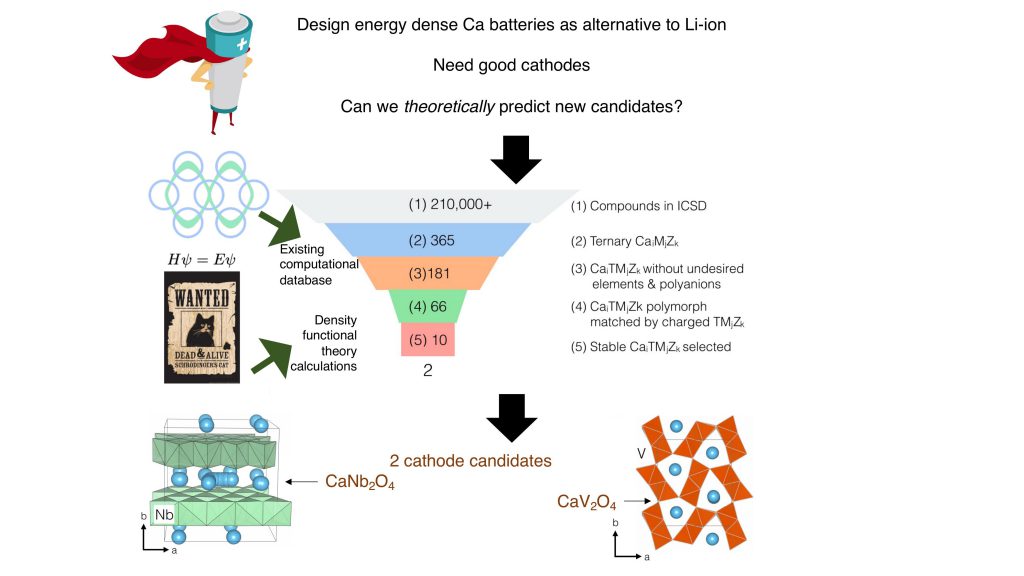
(Image: Gopalakrishnan lab)
The team screened for materials across all possible three-element systems with calcium – compounds composed of calcium, another redox-active transition metal, and an element from the oxygen series as a counter-ion. They found that only ten candidates were thermodynamically stable, and could potentially function as cathode materials. They then calculated some essential parameters that would govern the energy density and power of the battery, and zeroed in on two compounds, namely calcium vanadium oxide and calcium niobium oxide, that would make good cathode materials. “This is how screening studies work; there is some chemical intuition, some prior work that you use, and the new work you add to find new candidates,” says Sai.
From discovering transformational materials once every few centuries or even millennia, we are now in a position to develop such materials in just a matter of months
Abhishek’s lab is also involved in developing better thermoelectric materials – those that can convert waste heat to voltage – for more efficient energy generation. Recently, they developed a model that correlated thermal transport and electronic transport in a lattice, properties that were hitherto considered unconnected. “Electronic transport is much easier to calculate; we can do it in five to twenty minutes. But if you want to calculate the thermal conductivity of the material, it can take you one week easily,” he says. “Using electronic transport, now we can calculate the thermal transport easily.” This would be especially beneficial for computing some properties of thermoelectric materials which require both these parameters, and could accelerate the pace at which new thermoelectrics are discovered.
Abhishek is also interested in a class of transition metal-based compounds known as MXenes – these are two dimensional transition metal carbides or nitrides that are popular candidates for making batteries and sensors. His group developed “aNANt”, India’s first computational database for materials, with electronic and structural properties of nearly 25,000 MXenes in 2018. This could help researchers develop models for various applications, and help experimentalists pick the right materials for their specific applications, according to him.
Not all a bed of roses
Using computational tools and modelling to develop materials is not without limitations. Abhishek believes that the lack of high-quality reproducible data remains the biggest challenge in applying machine learning to the field of materials design. It is extremely important that we develop the infrastructure required to document and share the massive amount of data generated across the world, he says.
Moreover, the theoretical framework used for calculations at times comes with its own challenges. For instance, Sai’s group uses the density functional theory, a well-known quantum mechanical computational method, for their calculations. It is used to obtain an approximate solution to Schrödinger’s equation, a partial differential mathematical equation. “When we solve Schrödinger’s equation accurately, the properties you get are also very accurate. But here we’re solving it approximately; so the properties you get are also approximate, and there are limits to this approximation,” he admits. This theory is also scalable only for systems with a few hundred atoms, making it harder to study properties that emerge at a larger length scale. Moreover, knowing the properties of materials at the molecular level is even more challenging due to the quantum mechanical effects involved, adds Debiprosad. “If one really has to solve this problem, one would probably need quantum computers,” he adds, underscoring the importance of coupling quantum computing with solid mechanics for advancing computational materials science in the future.
In spite of these constraints, researchers across the globe continue to expand their toolkit with more powerful and multidisciplinary approaches, and we may very well be witnessing the green shoots of the next materials revolution. From discovering transformational materials once every few centuries or even millennia, we are now in a position to develop such materials in just a matter of months.
Karthika Kaveri Maiappan is a Master of Science student and a former science writing intern at the Office of Communications, IISc