AI is propelling science in unexpected directions, but it still needs scientists at the steering wheel
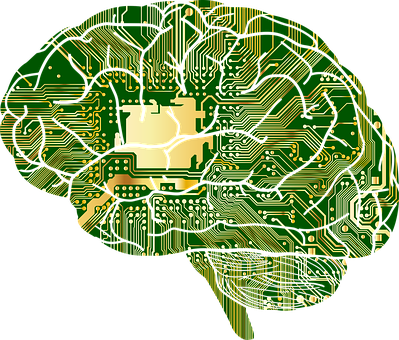
Artificial Intelligence, or AI, has penetrated every corner of our digital world. From aiding email servers identify spam, to helping websites recommend movies and GPS apps map out the quickest route through traffic, we increasingly depend on algorithms that enable computers to learn how to solve new problems. AI guided machines are rapidly becoming a powerful tool in the 21st century in every domain of human activity from farming to finance, automating assembly lines and self-driving cars, with revenues estimated to touch 50 billion dollars by 2020.
But more recently, AI has entered an arena beyond the immediate and practical demands of economics and industry and into the laboratories of almost every branch of science, detecting gravitational waves from colliding black holes, spotting early signs of Alzheimer’s disease, and designing new materials with novel properties. Leveraging the large amounts of data being generated by experiments around the world, scientists are now employing AI to ask questions that were formerly impossible to address and training them to recognise patterns in their data that are invisible to the human eye and difficult to anticipate.
More recently, AI has entered an arena beyond the immediate and practical demands of economics and industry and into the laboratories of almost every branch of science
Applications of AI in scientific research can be traced back to the end of the 20th century when experiments aided by rapid technological advances started generating data at an exponentially increasing rate, which were archived and shared freely over the Internet, ushering in the age of big data. But scientists are now faced with the daunting task of navigating this flood of information and are turning to AI to mine its depths.
Prateek Sharma, a professor of astrophysics at the Indian Institute of Science, talks about the Sloan Digital Sky Survey, a wide-angle telescope that collects optical data to create a detailed 3-dimensional map of the universe. Since it began operating in 2000 it has collected images of more than 3 million astronomical objects from the outermost limits of the observable night sky. “It is humanly impossible to look through each and categorise them,” says Sharma. “I know professors of astronomy who have switched entirely from astronomy to getting their hands dirty in big data.”
Astrophysicists and astronomers, who bear the unenviable responsibility of understanding the whole universe, aren’t the only scientists seeking refuge in AI. Abhishek Singh, a materials science researcher at IISc, talks about the Materials Genome Project – an initiative to discover new material structures through data-driven and machine-learning-assisted approaches. After a 2012 study published in Nature reported that “a machine-learning model outperformed traditional human strategies” in predicting the conditions for successfully synthesising a new material, Singh grasped the importance of AI in materials research and took the plunge. “After realising the power of AI in materials science, we formed a small group consisting of students and postdocs and started to develop machine learning-based models to accelerate the discovery of new functional materials.” AI refers to the field, and machine learning (ML) refers to the tools of designing computers than can act intelligently; they are frequently used interchangeably.
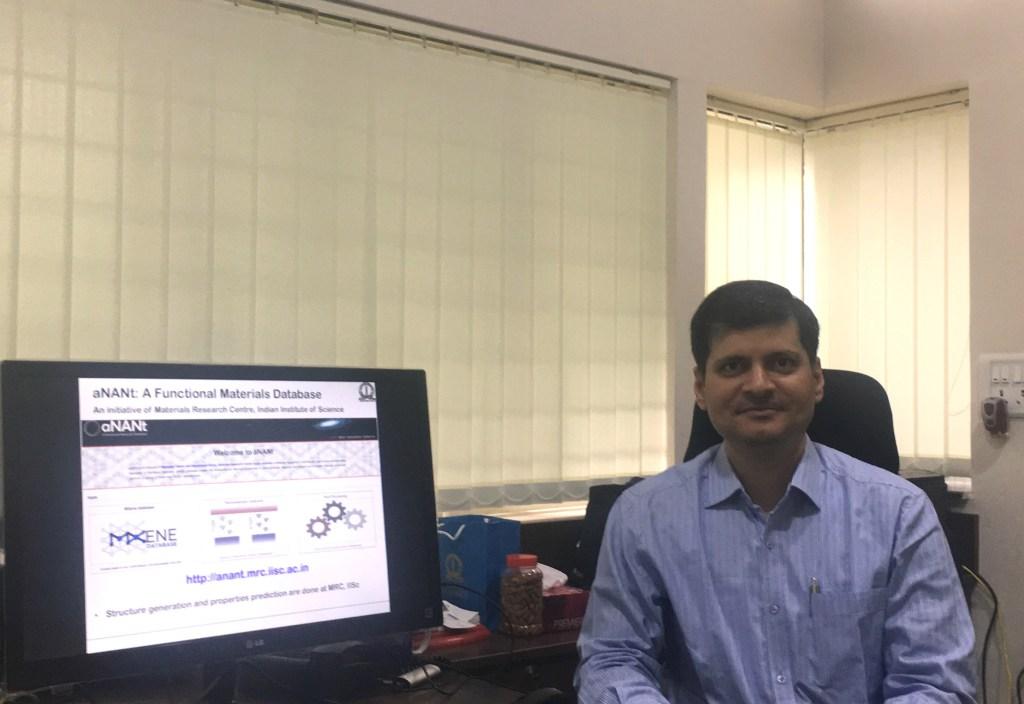
Sharma and Singh are among a wave of scientists who see promise in this new approach to employing machine intelligence for knowledge discovery in scientific research. What makes it possible to apply the same type of AI algorithm that can identify new astronomical objects in distant galaxies as well as predict the photoelectric properties of a new material is, in fact, a simple exploit that the human brain performs all the time: recognising patterns. Like a digital calculator multiplying two 16-digit numbers in a split second by using high-speed processors, AI is pattern recognition on steroids, enabling high-speed processors powering machines to mine vast oceans of information teeming with hidden patterns that hold the key to new discoveries, but which no individual can hope to swim alone or even together.
Broadly speaking, AI is the science that enables computers to learn how to solve problems to accomplish a specific goal, like recognising and classifying objects. But instead of explicitly programming the problem-solving steps into the computer, as was traditionally done, modern AI systems autonomously learn to solve problems by recognising patterns in data, simulating the learning mechanisms of the human brain. Neural networks, which are a simplified mathematical model of the brain, are one example of such an AI technique that helps machines learn patterns. A neural network is first trained to recognise images, of say animals, by presenting them with several labelled examples of different animals. The network parameters are tuned in the training process according to precise mathematical rules for optimally distilling the essential features of animals from the training data. If properly trained, when the neural network encounters a new unlabelled image of one such animal, it is able classify it correctly with high accuracy.
Modern AI systems autonomously learn to solve problems by recognising patterns in data, simulating the learning mechanisms of the human brain
Training a machine to recognise simple images may seem trivial, but even a simple class of objects like dogs can have infinitely many variations depending on colour, size, breed, and postures. The learning algorithms must learn to identify the regularities among different dog images – eyes, nose, whiskers, ears, tail, etc. – from the training data and associate the regular patterns with the labels while ignoring the details that are inessential. The engine that powers AI technologies comprises such learning algorithms that can extract these statistical regularities hidden in the data unique to each label or class.
The same general strategy works for any kind of training data. So long as they are appropriately labelled and large enough in numbers for the statistical regularities to be robustly computed, the algorithms can distil and extract these patterns. With properly trained AI, data such as astronomical images, material structures, and brain activity patterns can be classified as supernova or a gamma-ray burst, semiconductor or photodiode, Alzheimer’s patient or healthy.
Sridharan Devarajan, professor of neuroscience at IISc, is developing new tools for the early detection of Alzheimer’s Disease (AD), a devastating brain disorder with no known cure that destroys memory and cognitive abilities. AD is typically preceded by symptoms of dementia followed by abrupt decline of mental abilities. “It will be great if we can predict two years in advance if the disease is going to set in,” says Sridharan, “but the diagnosis of AD can be confirmed only post-mortem, making it challenging to diagnose before severe behavioural deficits onset.” Machine learning tools have proved indispensable in this effort.
To train his algorithms, Sridharan relies on large databases such as the Alzheimer’s Disease Neuroimaging Initiative (ADNI), which contains neurological data from over thousand participants, both healthy subjects and AD patients. By analysing the archived data of brain activities of patients collected over several years, machine learning algorithms are being trained to isolate and classify the patterns in brain activity that reliably signal the onset of AD. These symptomatic patterns can then be applied to diagnose at-risk patients who show similar brain activity patterns before the symptoms start manifesting behaviourally, when it’s too late to intervene. Early detection may allow medical interventions and lifestyle changes that are known to delay its onset.
Such datasets are essential in AI for training the learning algorithms. Many scientists are now routinely archiving and sharing their experimental data freely over the web, ushering in a new era of collaborative research on a global scale. Singh has started such an initiative at the Materials Research Centre called aNANt, an online repository of functional materials freely available to scientists anywhere in the world. The still-growing database contains information about structures and electronic properties of over 15,000 computationally designed materials. aNANt joins similar databases in the United States, Europe and Asia as a part of the Materials Genome Initiative. The total number of such materials adds up to more than a billion worldwide, and can be used to train machine learning models anywhere on the planet for predicting the properties of new structures. “Tomorrow, if you come up with a completely new material and you don’t know anything about it, if you just put its features into these models, it will tell you if it’s a good photo-catalyst or a good electronic material or a good sensor.”
Sometimes, however, there’s a dearth of experimental data for problems that are still in the early stages of research, as in the case of gravitational wave detection. Gravitational waves, first observed in 2016, are perturbations in the fabric of space-time that are caused by catastrophic events billions of light years away, such as the collisions of massive black holes or the merger of neutron stars, both extraordinarily heavy and dense objects confined in a small region of space. The precise signal of a gravitational wave detected on Earth due to such a collision depends on many factors. Sharma explains: “You have observed a signal, but there are so many parameters to calculate. What are the masses, the plane’s inclination, what are the spin directions?” To identify these parameters, scientists are now using AI. But instead of analytically working out the solutions to the to their equations, which requires tedious calculations, astrophysicists are simulating thousands of such collisions on supercomputers to generate surrogate data with varying masses, spins and planes of orbits for training their learning algorithms. Once the patterns are learned, they can then be used to identify new gravitational wave signals and immediately predict the locations, spins and masses of the black holes. Astronomers may soon benefit from trained algorithms operating continuously without sleep or break to classify images detected by telescopes.
Despite the growing enthusiasm and initial success of AI in science, the progress hasn’t come without concerns. Stephen Hawking famously said, “The development of full artificial intelligence could spell the end of the human race.” Some scientists echo the fear that AI could diminish the role of scientists and strip science of human creativity, mechanising research and fundamentally altering the spirit of scientific discovery. Others, however, believe that the fears are premature, if not entirely unfounded. While AI is still in its infancy and expected to become significantly more powerful with better hardware, faster processors and improved algorithms, there are obstacles that may be too high for even fully developed AI to scale all by itself. The true potential of AI can be realised not by blindly applying machine learning algorithms on scientific data but by understanding how the algorithms work in conjunction with scientific insights acquired through rigorous education and experience.
Any sufficiently large dataset will have a plethora of hidden patterns like faces in clouds, but most of them are unlikely to be scientifically meaningful. For the learning algorithms to successfully identify the relevant and meaningful patterns and provide real scientific insight, the data has to be organised carefully, labelled appropriately and presented methodically to the AI algorithms and that requires understanding of the research context and question. Moreover, even when the datasets are optimally organised, AI systems cannot recognise meaningful patterns that they haven’t been trained for. Recognising novel phenomena cannot be achieved without human imagination and an ability to conceive and expect new possibilities and scenarios.
Recognising novel phenomena cannot be achieved without human imagination and an ability to conceive and expect new possibilities and scenarios
Furthermore, AI algorithms require well-defined goals and in science the goals depend intimately on the question being posed. For example, the goal of a clinical researcher may be to answer the question: what are the brain activity patterns of Alzheimer’s disease in a specific brain area? AI has no ability to formulate even simple questions without which patterns have no intrinsic value. Sridharan sums up this limitation of AI succinctly: “With AI you may be able to extract knowledge, but when it comes to extracting meaning and understanding the bigger picture, I doubt if AI can do that.”
Werner Heisenberg, father of the uncertainty principle, once said, “What we observe is not nature itself, but nature exposed to our questioning.” Perhaps, with the task of identifying patterns and extracting knowledge delegated to machines, the human mind will be free to devote itself more fully to asking new questions that are currently inconceivable, perhaps even unreasonable, but which could become seeds of new discoveries and bigger adventures.
Ranit Sengupta was a postdoctoral researcher in The Cognition Lab directed by Sridharan Devarajan at the Center for Neuroscience, IISc. He currently works as a freelance writer based out of Bangalore.